Python Program to Implement the k-Nearest Neighbour Algorithm
Exp. No. 9. Write a program to implement k-Nearest Neighbour algorithm to classify the iris data set. Print both correct and wrong predictions. Java/Python ML library classes can be used for this problem.
K-Nearest Neighbor Algorithm
Training algorithm:
- For each training example (x, f (x)), add the example to the list training examples Classification algorithm:
- Given a query instance xq to be classified,
- Let x1 . . .xk denote the k instances from training examples that are nearest to xq
- Return
- Given a query instance xq to be classified,
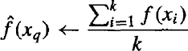
- Where, f(xi) function to calculate the mean value of the k nearest training examples.
Data Set:
Iris Plants Dataset: Dataset contains 150 instances (50 in each of three classes) Number of Attributes: 4 numeric, predictive attributes and the Class.
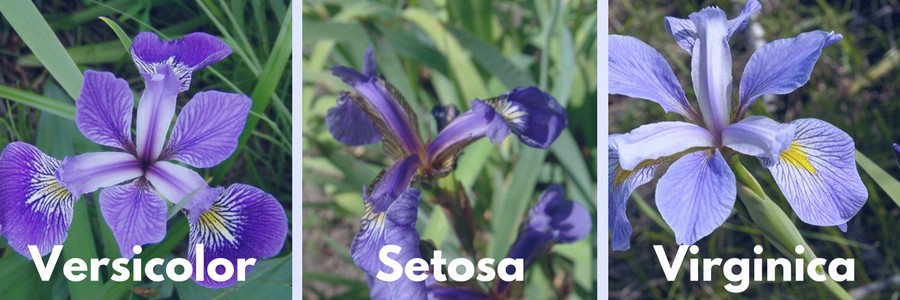
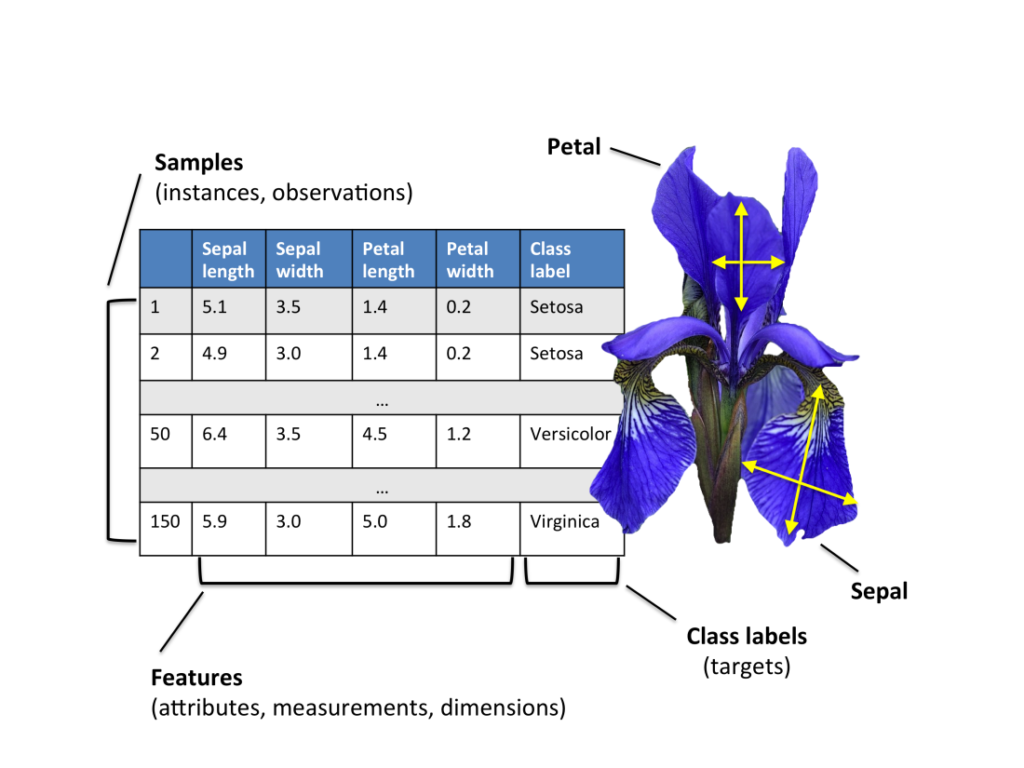
Sample Data
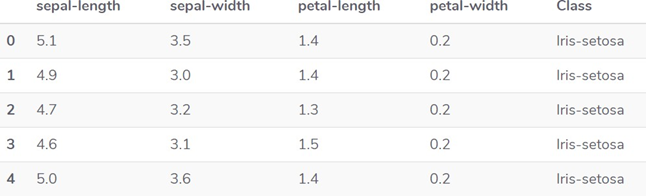
Click Here to Download Iris Dataset
Python Program to Implement and Demonstrate KNN Algorithm
import numpy as np import pandas as pd from sklearn.neighbors import KNeighborsClassifier from sklearn.model_selection import train_test_split from sklearn import metrics names = ['sepal-length', 'sepal-width', 'petal-length', 'petal-width', 'Class'] # Read dataset to pandas dataframe dataset = pd.read_csv("9-dataset.csv", names=names) X = dataset.iloc[:, :-1] y = dataset.iloc[:, -1] print(X.head()) Xtrain, Xtest, ytrain, ytest = train_test_split(X, y, test_size=0.10) classifier = KNeighborsClassifier(n_neighbors=5).fit(Xtrain, ytrain) ypred = classifier.predict(Xtest) i = 0 print ("\n-------------------------------------------------------------------------") print ('%-25s %-25s %-25s' % ('Original Label', 'Predicted Label', 'Correct/Wrong')) print ("-------------------------------------------------------------------------") for label in ytest: print ('%-25s %-25s' % (label, ypred[i]), end="") if (label == ypred[i]): print (' %-25s' % ('Correct')) else: print (' %-25s' % ('Wrong')) i = i + 1 print ("-------------------------------------------------------------------------") print("\nConfusion Matrix:\n",metrics.confusion_matrix(ytest, ypred)) print ("-------------------------------------------------------------------------") print("\nClassification Report:\n",metrics.classification_report(ytest, ypred)) print ("-------------------------------------------------------------------------") print('Accuracy of the classifer is %0.2f' % metrics.accuracy_score(ytest,ypred)) print ("-------------------------------------------------------------------------")
Output
sepal-length sepal-width petal-length petal-width 0 5.1 3.5 1.4 0.2 1 4.9 3.0 1.4 0.2 2 4.7 3.2 1.3 0.2 3 4.6 3.1 1.5 0.2 4 5.0 3.6 1.4 0.2 ------------------------------------------------------------------------- Original Label Predicted Label Correct/Wrong ------------------------------------------------------------------------- Iris-versicolor Iris-versicolor Correct Iris-virginica Iris-versicolor Wrong Iris-virginica Iris-virginica Correct Iris-versicolor Iris-versicolor Correct Iris-setosa Iris-setosa Correct Iris-versicolor Iris-versicolor Correct Iris-setosa Iris-setosa Correct Iris-setosa Iris-setosa Correct Iris-virginica Iris-virginica Correct Iris-virginica Iris-versicolor Wrong Iris-virginica Iris-virginica Correct Iris-setosa Iris-setosa Correct Iris-virginica Iris-virginica Correct Iris-virginica Iris-virginica Correct Iris-versicolor Iris-versicolor Correct ------------------------------------------------------------------------- Confusion Matrix: [[4 0 0] [0 4 0] [0 2 5]] ------------------------------------------------------------------------- Classification Report: precision recall f1-score support Iris-setosa 1.00 1.00 1.00 4 Iris-versicolor 0.67 1.00 0.80 4 Iris-virginica 1.00 0.71 0.83 7 avg / total 0.91 0.87 0.87 15 ------------------------------------------------------------------------- Accuracy of the classifer is 0.87 -------------------------------------------------------------------------
Summary
This tutorial discusses how to Implement and demonstrate the k-Nearest Neighbour Algorithm in Python. If you like the tutorial share it with your friends. Like the Facebook page for regular updates and YouTube channel for video tutorials.